Before we delve into the fascinating evolution of AI in robotics, I encourage you to first explore our main blog post. It lays the groundwork for understanding how artificial intelligence has become a pivotal force in advancing robotics. From the early days of simple machines to today’s sophisticated AI-driven systems, the journey is both intriguing and inspiring.
Read the full context here: AI Robotics Journey Intro
From now on, we will be referring to this series as the Artificial Intelligence for Robotics (AIR) Journey.
Without further ado, let’s take a closer look at the remarkable journey of AI in robotics, exploring key milestones and breakthroughs that have shaped this dynamic field.
Expert: Konstantin
Interviewer: Robi
Could you please introduce yourself and briefly describe your experience in the field of AI and robotics?
My name is Konstantin, and I'm the CEO and one of the founders of ARTI. I studied Information and Computer Engineering (earlier called Telematics) at the Graz University of Technology and chose AI and robotics for my master's classes. Back in those days, these topics were separate, but I felt that both disciplines worked perfectly together. Since then, I have continued to immerse myself in learning both disciplines, driven by both business-related inquiries and personal interest.
Let's start from the beginning. How would you describe the early stages of robotics and the initial role of AI in this field?
Robotics and AI have a fairly long history and have shaped each other along the way. Scientists believe that true intelligence can only emerge through interaction with the real world. Many early-stage robots were equipped with cameras, primarily due to the lack of other technologies. People often compared these cameras to human vision, striving to achieve similar performance. Artificial intelligence, as we know it today, was limited in many ways—lack of computational power, limited understanding of brain functions, etc. Despite these limitations, some impressive results were achieved during that time.
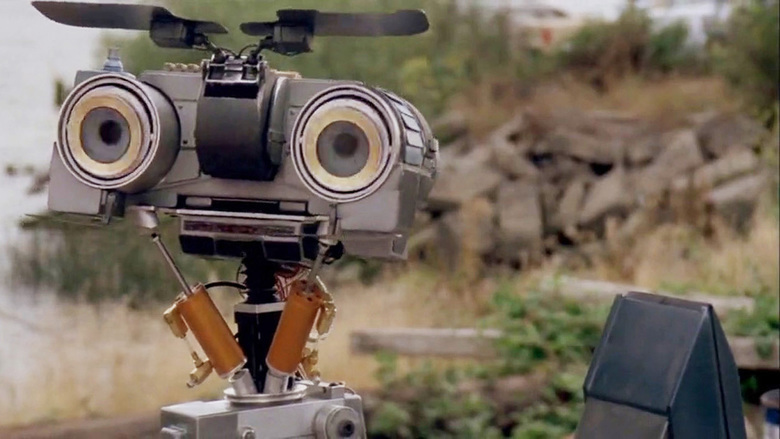
What do you consider to be the key milestones in the evolution of AI in robotics? Could you share some pivotal moments or breakthroughs?
When discussing the evolution of AI in robotics, Stanford University must be mentioned. A groundbreaking project from the 1960s was Shakey, the first mobile robot to intelligently perceive its environment, draw conclusions, and act accordingly. Although the environments and conclusions were relatively simple, significant advancements were made in understanding computer vision, manipulation, and pathfinding. In industrial applications, robots were heavily pre-programmed to perform unpleasant tasks or tasks requiring high precision quickly. For robots to be effective in the real world, they need to understand a complex and diverse environment. This is why Stanley, the self-driving vehicle that successfully completed the course of the DARPA Grand Challenge, was also a noteworthy milestone. Intelligent robots suddenly became a viable option for industrial applications on a larger scale.
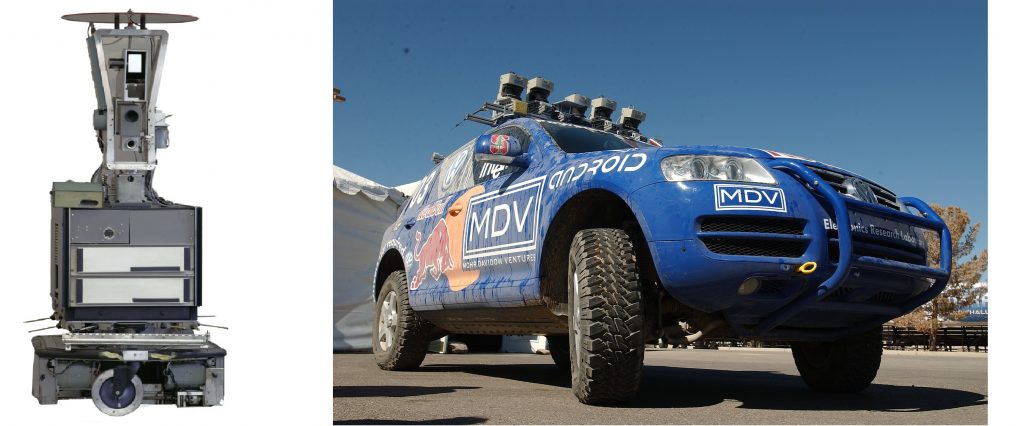
How have advancements in technology, such as computing power and algorithms, impacted the development of AI in robotics over the years?
The new wave of AI has revolutionized algorithms for deep neural networks and large-scale data processing. These advancements were further accelerated by lightning-fast processing speeds and very affordable data storage. Engineers demonstrated that, with sufficient data and processing speed, neural networks can pursue high-level goals. Notably, Nvidia has been at the forefront of this research, teaching their self-driving car, End-to-End, by inputting camera footage and steering commands, which the AI replicated in an impressive manner. It was believed that AI could be trained by simply showing and demonstrating tasks, provided there was enough data. However, this approach turned out to be a dead end for complex tasks.
Find out more about Nvidia End-to-End Deep Learning for Self-Driving:
https://developer.nvidia.com/blog/deep-learning-self-driving-cars/
In your view, what were the major challenges in integrating AI into robotics, and how have these been addressed over time?
Besides the supervised learning approach, where scientists encountered the problem of 'not enough data,' the alternative of reinforcement learning became more promising. The concept behind reinforcement learning is relatively simple: the agent (or in our case, the robot) attempts to find the best solution or behavior until it masters the task. However, it became apparent that this requires a significant amount of time and/or robots to train for the necessary duration. Meanwhile, generative AI improved more than ever before. You are likely familiar with this approach through ChatGPT for text generation or DALL-E for image creation. The same idea can be applied to generate additional training data for robots. As a new frontier, companies like OpenAI, Nvidia, and others have been successful in teaching robots inside simulations. A major milestone was the solving of the Rubik's Cube with a robotic hand from scratch.
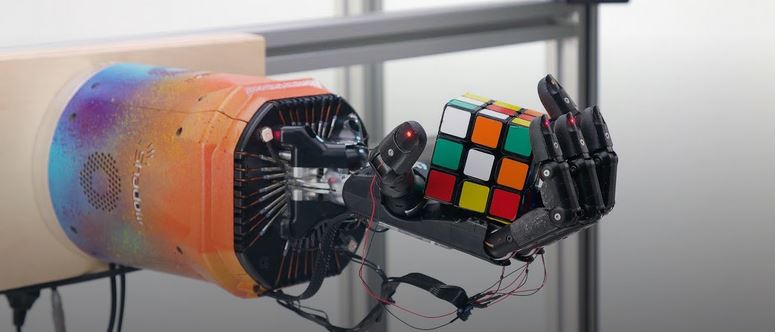
How would you describe the current state of AI in robotics? What are the most impressive applications you've seen recently?
In a single word, I would describe it as 'Evolving.' The advancements in robotics are indeed very impressive, yet there remains a fundamental contradiction. On one hand, robots are capable of performing more advanced tasks than ever before; on the other hand, it's challenging to achieve high precision in specific tasks. Balancing flexibility and precision in task solving appears to be a difficult feat. Highly impressive solutions like Atlas from Boston Dynamics or ANYmal from ANYBotics are ushering in the next era of robotics. Both systems are pioneers in control engineering, demonstrating exceptional performance in acrobatic actions. If you haven't seen them in action yet, I encourage you to do so. Proving a robot for our daily houshold work is yet out of reach. Developing a robot capable of handling our daily household tasks remains an elusive goal.
Video of Atlas – Boston Dynamics Parkour
Video of ANYmal from ANYBotics
How significant is the role of data in the advancement of AI-driven robotics? Can you elaborate on the importance of data quality and quantity?
Data transforms into knowledge. As AI models grow larger and the complexity of tasks increases, the need for more data to create a useful solution becomes imperative. It's challenging to identify what might be missing within the training dataset. Over the last decade, engineers have realized that data quality and its consistency are not only crucial for solving a task but also for enhancing performance. The term 'black box' emerged due to the lack of understanding regarding the model architecture and the data used for training. This lack of insight led to AI learning irrelevant features, such as shadows, or exhibiting strong biases towards certain objects. The significance of data quality and quantity is evident in today's large language models, where you can download already trained models but not the actual training data, which could reveal flaws in usage.
As AI in robotics has evolved, what ethical and social implications have emerged? How should the industry address these concerns?
With the integration of AI, robotics gains access to a broader world, and robots are set to become a part of our daily lives. Many people are already utilizing autonomous lawn mowers and vacuum cleaning robots to automate daily chores. On a larger scale, self-driving vehicles, delivery robots, and similar technologies will soon become more common in public spaces. In the past, robots were often confined to cages to prevent accidents, but as robots begin to work alongside people (including those without specialized training), the industry must delve deeper into understanding the long-term social implications. Intelligent robots need to better comprehend social aspects, scenarios, and the requirements for addressing human-machine interaction across all generations. Robots should promote social inclusiveness.
Finally, based on the evolution you've witnessed, what predictions can you make about the future of AI in robotics? What are the next big things we should look out for?
For the first time in human history, AI is becoming the most disruptive technology we have ever witnessed, and it will lead to drastic changes across numerous domains. In 2023, we have witnessed the emergence of multimodal AIs, capable of hearing, speaking, and seeing, with development showing no signs of slowing down. The field is advancing more rapidly than any expert had predicted. Intelligent robots serve as the perfect example of multimodal problems, requiring the ability to sense, think, and act within their environment. Who knows, perhaps we are on the verge of introducing a RobotGPT (comparable to ChatGPT), capable of gracefully solving many tasks we could only dream of. As an artist at ARTI, my job is to ponder why it isn't already a reality.
Stay tuned as we continue to explore the world of AI in robotics in our upcoming series. Thank you for joining us on this journey of discovery!